The Benefits of Continual Learning for the European Steel Industry
In previous articles, we discussed how the ALCHIMIA project will implement federated learning and transfer learning solutions to accomplish our goal of transforming the European steel industry. In the final article of this series, we will look at continual learning, the third feature that will ensure our machine learning solutions remain relevant to the industry for a long time to come.
Continual learning aims to combat a fundamental fact of nature: everything changes, nothing stays the same. When a machine learning model is trained, it is ideally done with the most up to date data available. The resulting model reflects the relationships between important factors in the environment and the variable it is trying to predict. That is, it has learned to predict the status quo.
But while machine learning models can capture incredibly complex and nuanced relationships, they cannot see the future nor understand the world around them. Should something about the world change, they have no way of reacting to this change. A perfect example of such a change was the COVID-19 pandemic. The pandemic caused a near total disruption of day-to-day life, rendering old models next to useless. Imagine a model that predicts the level of traffic on a given day at a given time. That model would have continued to predict high traffic on weekdays throughout the pandemic, even as people self-isolated and the streets were empty.
This is an extreme example, and changes need not be so dramatic for continual learning to be applicable. Smaller events can have similar effects: a change in a company or government policy, the arrival of a new competitor in the market, the discovery of a more efficient process. All these change the status quo, and machine learning models based on the old way of doing things need to play catch up.
Enter: continual learning. Continual learning is the practice of developing models that can adapt over time to changing demands by, you guessed it, continuously learning from the latest data available. Instead of a set-and-forget approach, continual learning ensures models are constantly updated to maintain the performance that made them so effective when they were first trained.
The steel industry is the perfect environment to reap the benefits of this approach. Particularly given the pressure to combat climate change and reduce the emission of greenhouse gases, the processes and practices of the industry are likely to change from one day to the next, in small ways and in large. Research may lead to the discovery of new and more effective approaches; policy changes may force small or large shifts in industry practices; and the demand for steel may fluctuate as the global economy develops and evolves. Machine learning models must be constantly updated so that they benefit from the learning of the past, while keeping up with the changes in the present.
Continual learning and federated learning go hand in hand, as the global machine learning model is constantly refined with information from participating plants. Combine this with transfer learning, and a relevant, accurate and customized machine learning model is always at the fingertips of Europe’s steel plants.
Footnotes
Article on federated learning: https://alchimia-project.eu/the-benefits-of-federated-learning-for-the-european-steel-industry/
Article on transfer learning: https://alchimia-project.eu/the-benefits-of-transfer-learning-for-the-european-steel-industry/
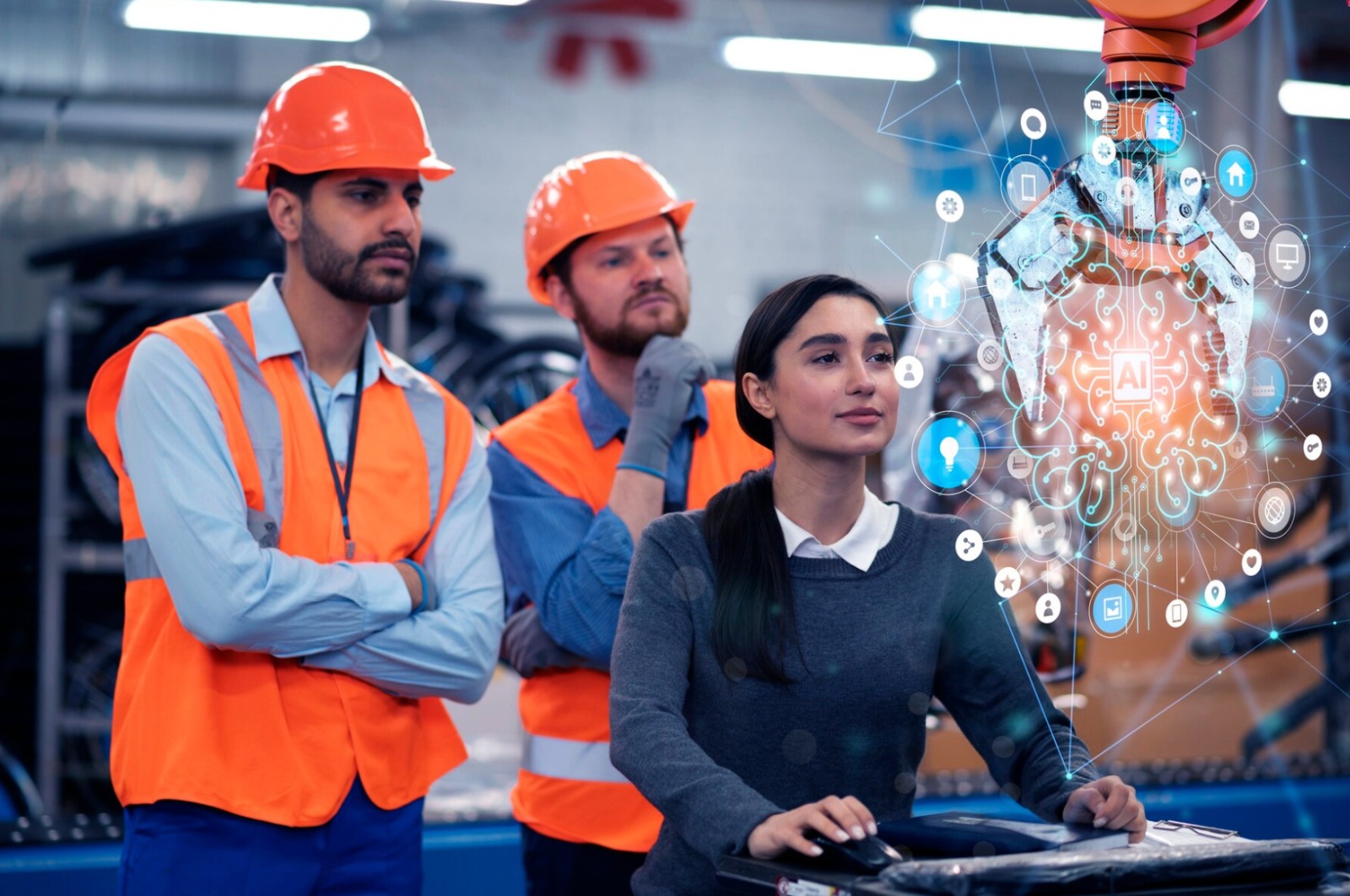