Smarter AI for Industry: ALCHIMIA Advances Its Federated Learning Framework
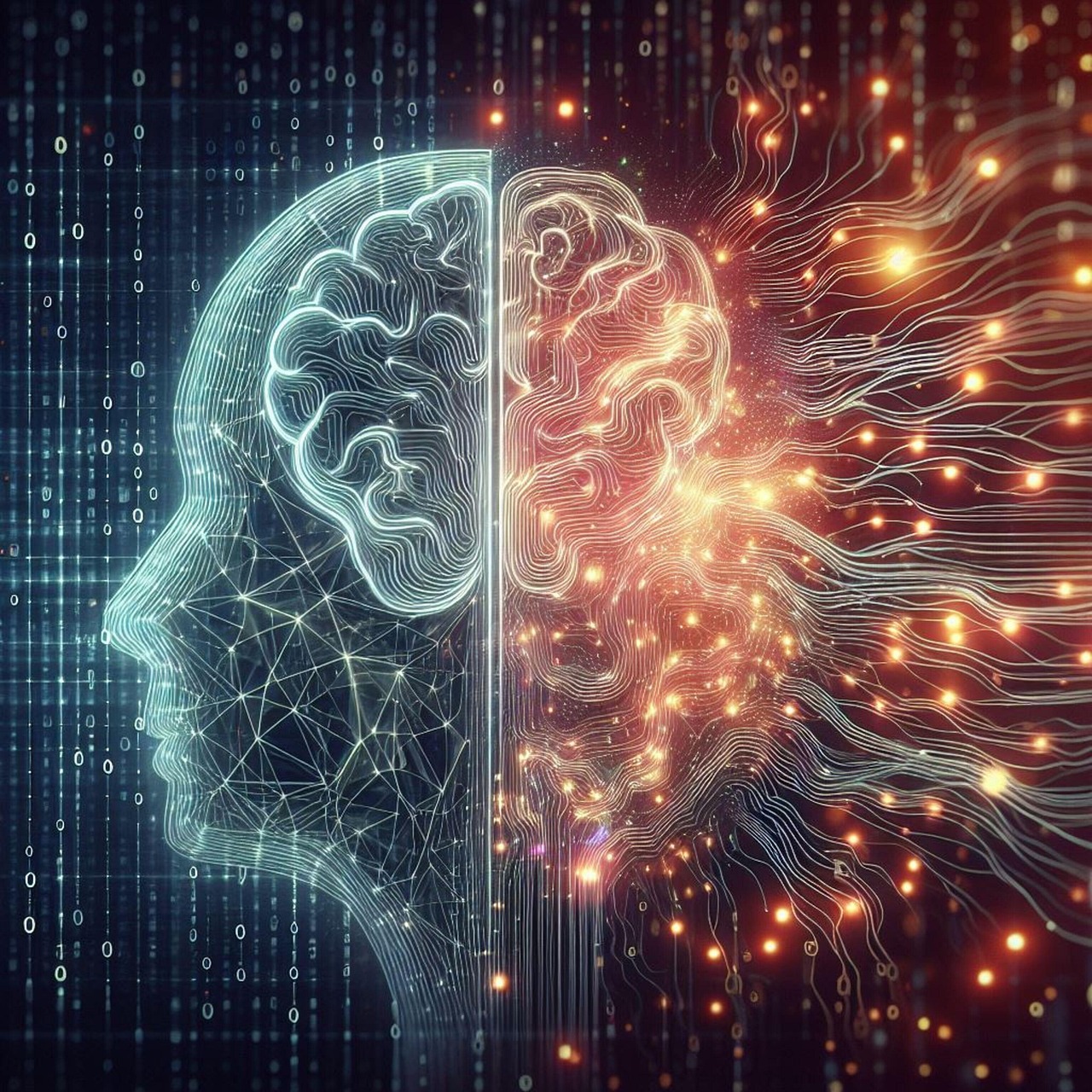
How can Artificial Intelligence adapt to the real-world complexity of industrial manufacturing? Within the ALCHIMIA research project, Atos has been tackling this challenge head-on by developing a custom Federated Learning (FL) framework. Now, with the delivery of the second (and final) version of Deliverable D3.1, the team reports major advancements in this core component of the project.
Why Federated Learning Matters
The development of this framework is a key objective for ALCHIMIA, as FL can offer significant benefits to the project’s industrial partners – two enterprises in the metallurgy sector, each with multiple production plants. In this multi-plant setup, sites within the same company can benefit from collaborative training of Machine Learning (ML) models, all while preserving data privacy.
To meet ALCHIMIA’s specific requirements, a novel Federated Learning framework is being developed from scratch. As explained in D3.1, existing solutions from the literature were not suitable due to the need for a fully configurable and adaptable system. Modularity and scalability are essential, especially as the framework will integrate not only classical FL features but also advanced capabilities like Continual Learning (CL) and Transfer Learning (TL).
Beyond FL: Enabling Continual and Transfer Learning
- Continual Learning (CL):
This feature is vital in industrial environments such as ALCHIMIA’s. It allows deployed ML models to be continuously monitored for performance degradation—triggering automatic retraining to adapt to new data patterns. This significantly extends model lifespan and avoids the inefficiency of using underperforming models in production.
- Transfer Learning (TL):
Given that the two industrial partners operate similar yet not identical processes, TL helps explore whether some features or knowledge can be reused across organizations. This not only boosts efficiency but also promotes shared innovation across different industrial setups.
What’s New in This Version?
The deliverable outlines how Atos has extended the FL framework to integrate these capabilities—combining the strengths of FL, CL, and TL to fully address ALCHIMIA’s needs. It also summarizes the ML models developed in D4.1, highlighting those selected as potential candidates for FL.
Additionally, it explains how ALCHIMIA’s architecture (from D2.2) has been translated into a decentralized MLOps setup, ready to be deployed across partner infrastructures (ATOS, CELSA, and FDT) using distributed hardware.
Real-World Testing: Promising Results
One of the key experiments featured in the deliverable is the federation of CELSA’s Ladle Furnace (LF) models. The results show that federated models can actually improve overall performance, demonstrating the effectiveness of WP3 technologies when applied to real industrial use cases.
KPI Achieved: FL Framework Ready for Action
With the addition of key features, the delivery of this version of the FL framework successfully fulfils the related KPI: a prototype of the ALCHIMIA Federated Learning and Continual Learning framework. This milestone sets the stage for smarter, scalable, and privacy-preserving AI adoption in the manufacturing sector.